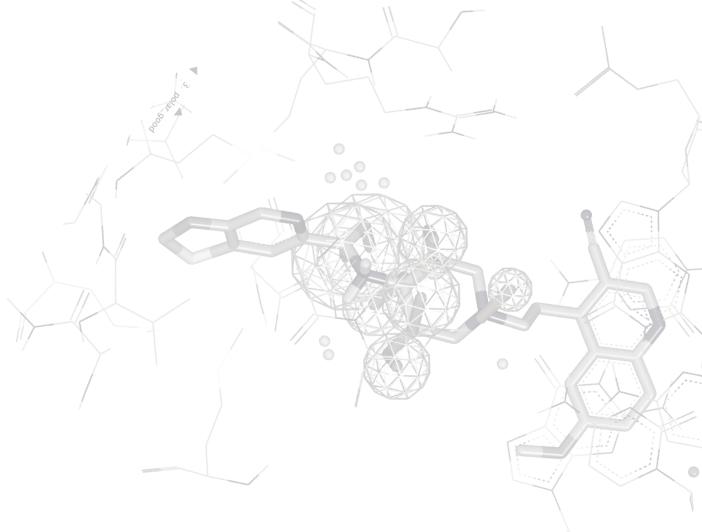
3D-QSAR
The OpenEye's 3D Quantitative Structure–Activity Relationship tool (3D-QSAR) creates models for predicting binding affinity based on descriptors derived from the 3D conformers of molecules. It uses molecular similarity between aligned ligands as the primary descriptor, combining multiple models with different similarity descriptors and machine learning techniques. The model's prediction is a consensus of individual model predictions.
Contact us to learn how you can gain new therapeutic opportunities using OpenEye's 3D-QSAR.
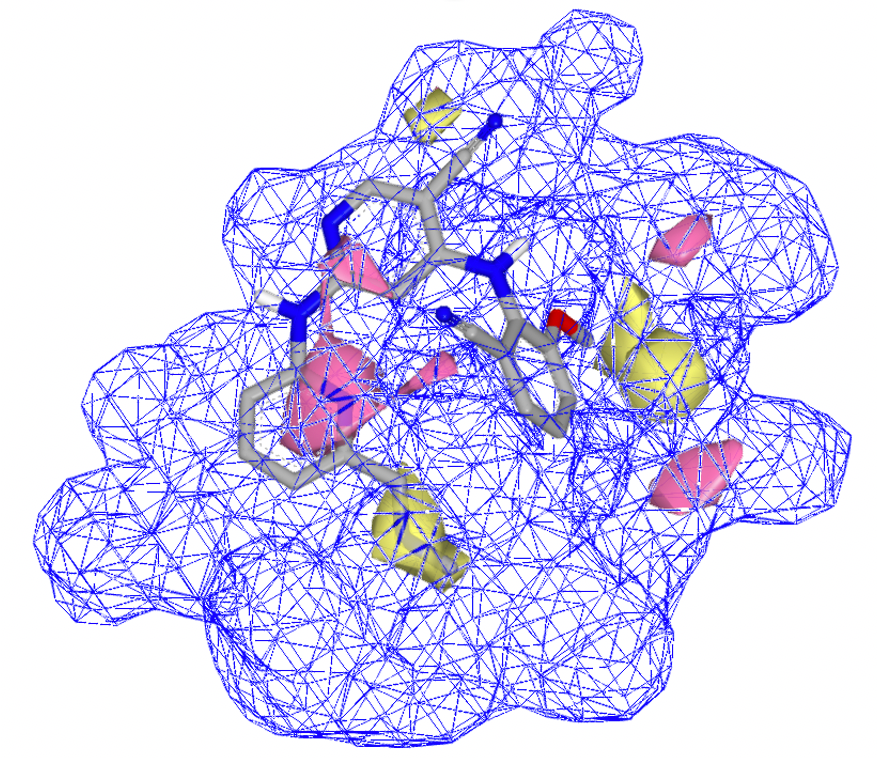
Features
-
Cutting Edge. Descriptors based on our industry leading OpenEye 3D shape and electrostatic tools (ROCS and EON)
-
Robust. Prediction provided as consensus of multiple models
-
Flexible. Utilizes 3D conformers provided by users or generated using OpenEye tools
-
Confidence. Prediction confidence based on domain of applicability
-
Interpretable. Model interpretation indicating favorable sites of functional groups
Inspiring new ideas through the interpretation of visual models
A big challenge for 3D-QSAR is that it can be difficult to interpret a model. OpenEye’s 3D-QSAR model helps scientists interpret regions within the active site, indicating preferences for specific features
3D-QSAR doesn't know or impute anything about the receptor/binding site, it picks out strongly contributing features in the model. These interpretations can inspire new ideas and make the model a useful part of a generative design cycle.
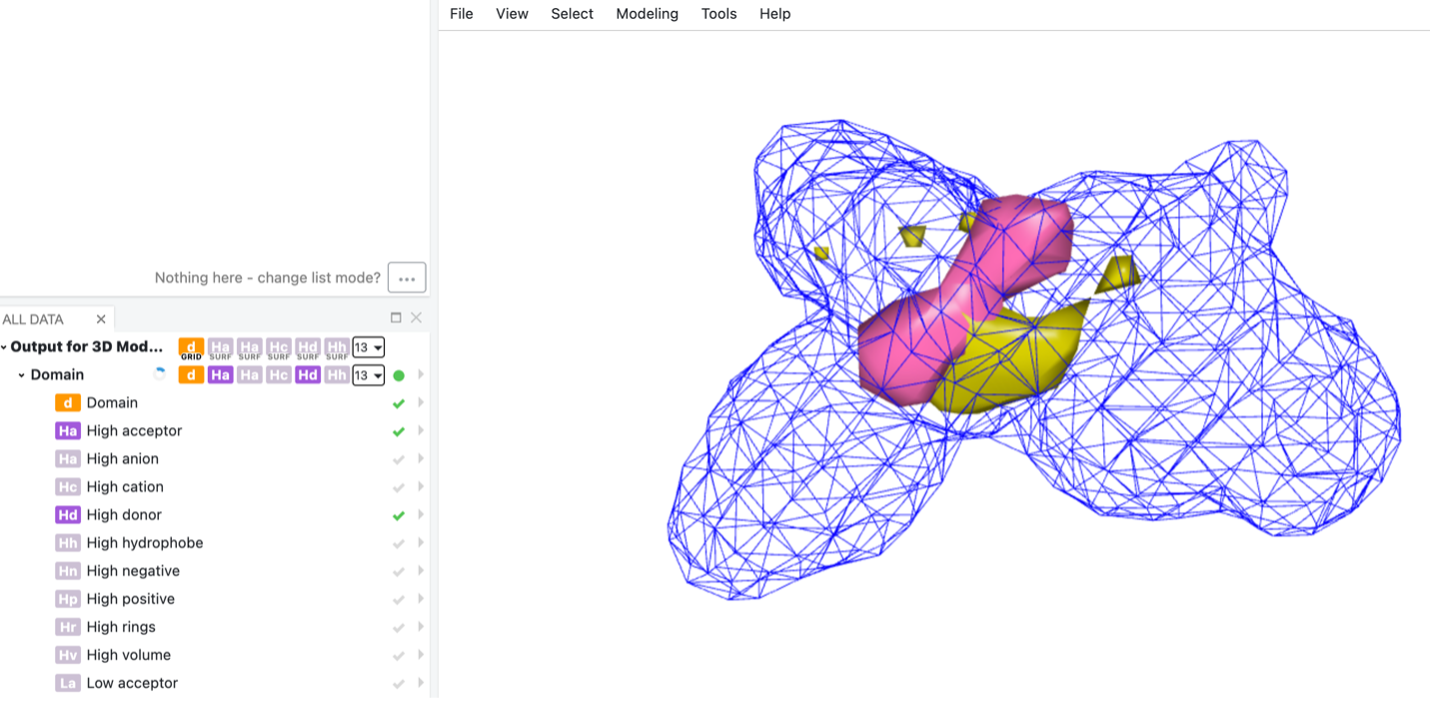
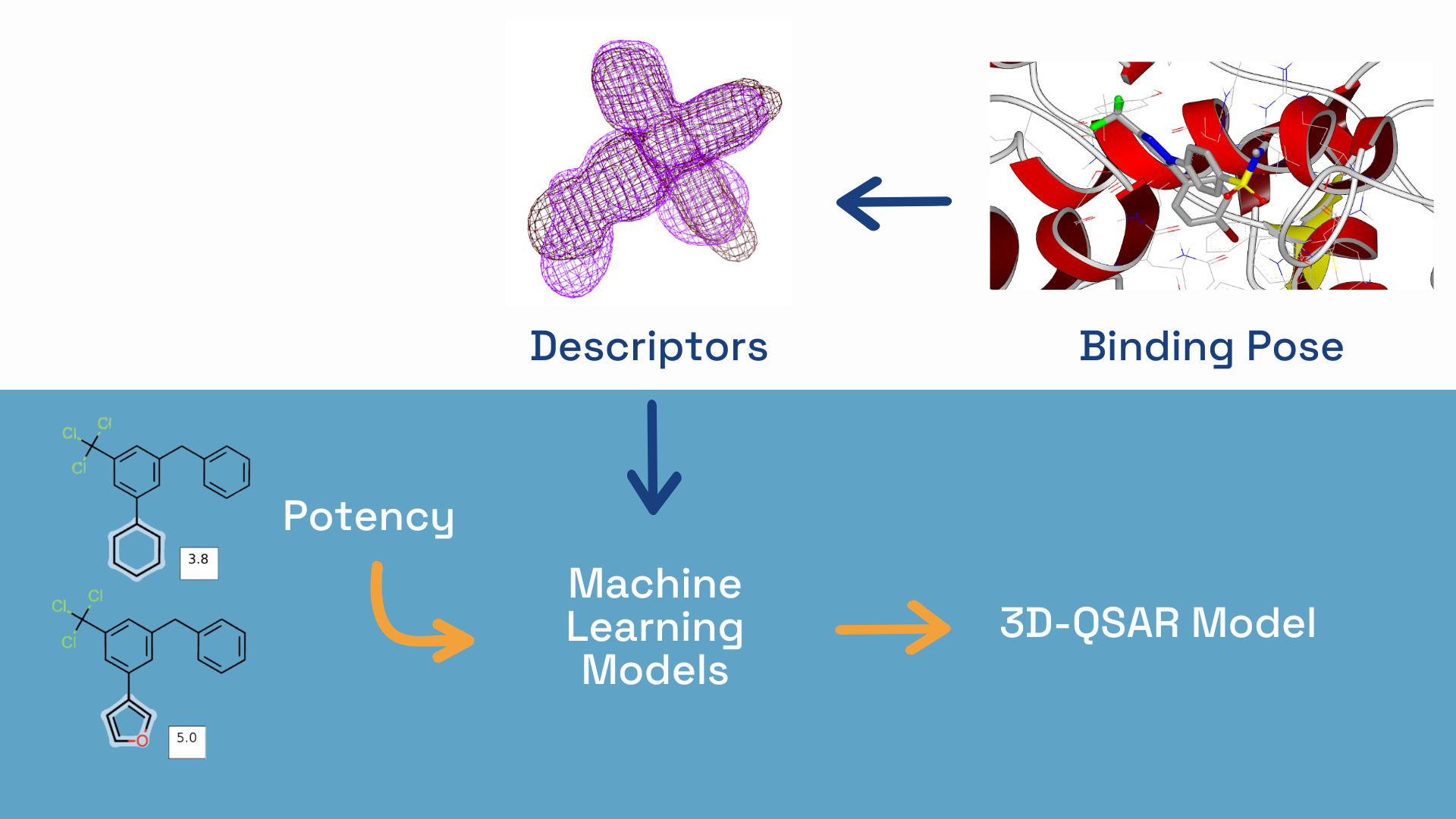
Innovative science designed in collaboration with industry partners
We developed the 3D-QSAR tool in partnership with a premier AI drug discovery company. Leveraging OpenEye’s cutting-edge expertise in 3D shape and electrostatics, the 3D-QSAR method provides drug discovery scientists with results that are interpretable and actionable for use in drug discovery.
-
The 3D-QSAR tool uses the well-validated ROCS® molecular shape- and color-based similarity and the more physically rigorous shape and electrostatic potential similarity from EON. Besides the ROCS- and EON-based similarity descriptors, 2D circular fingerprint (FP) based similarity is also included in some of the individual 3D models.
-
Two distinct machine learning approaches, namely kernel Partial Least Squares (kPLS) and Gaussian Process Regression (GPR), are used for model building. These methods are selected for their suitability for building models with small datasets, which is the case for potency models.
-
OpenEye’s 3D-QSAR offers a cost-effective approach to predict potency or binding affinity. Compute costs may vary depending on whether conformer generation is needed. Our tests average tens of dollars for a typical dataset of hundreds of molecules.
-
Refined 3D-QSAR Method and Consensus Model: The 3D-QSAR approach for deriving molecules now substantially outperforms traditional queries for the most potent molecules. The Consensus Model for the 3D-QSAR Floe package has been updated to a weighted model, which averages all included models, with 2D-GPR as the baseline. This new weighted model consistently delivers superior results compared to the previous unweighted model.
Learn More
3D QSAR Model for Binding Affinity Prediction. Shyamal K. Nath, Jingyi Chen, Ali Mozaffari and Riddhish Pandharkar. Ninth Joint Sheffield Conference on Chemoinformatics, University of Sheffield, UK, June 2023.
New 3D-QSAR Model for Binding Affinity Prediction. Ali Mozaffari, Jingyi Chen, Shyamal K. Nath. ACS Fall Meeting, San Francisco, CA, August 2023.
References
- Comparison of Shape-Matching and Docking as Virtual Screening Tools. Hawkins, P.C.D., Skillman, A.G., Nicholls, A., J. Med. Chem., 2007, 50, 74.
- The Use of Three-Dimensional Shape and Electrostatic Similarity Searching in the Identification of a Melanin-Concentrating Hormone Receptor 1 Antagonist S. Muchmore, A. J. Souers, I. Akritopoulou-Zanze, Chem. Biol. Drug Des., 2006, 67, 174.
RESOURCES
Glimpse the Future through News, Events, Webinars and more
New Year’s 2025 Fireside Chat with Anthony Nicholls
Cadence Announces New Life Sciences Leadership
Orion® Molecular Design Platform Lunch & Learn Feb 11, 2025
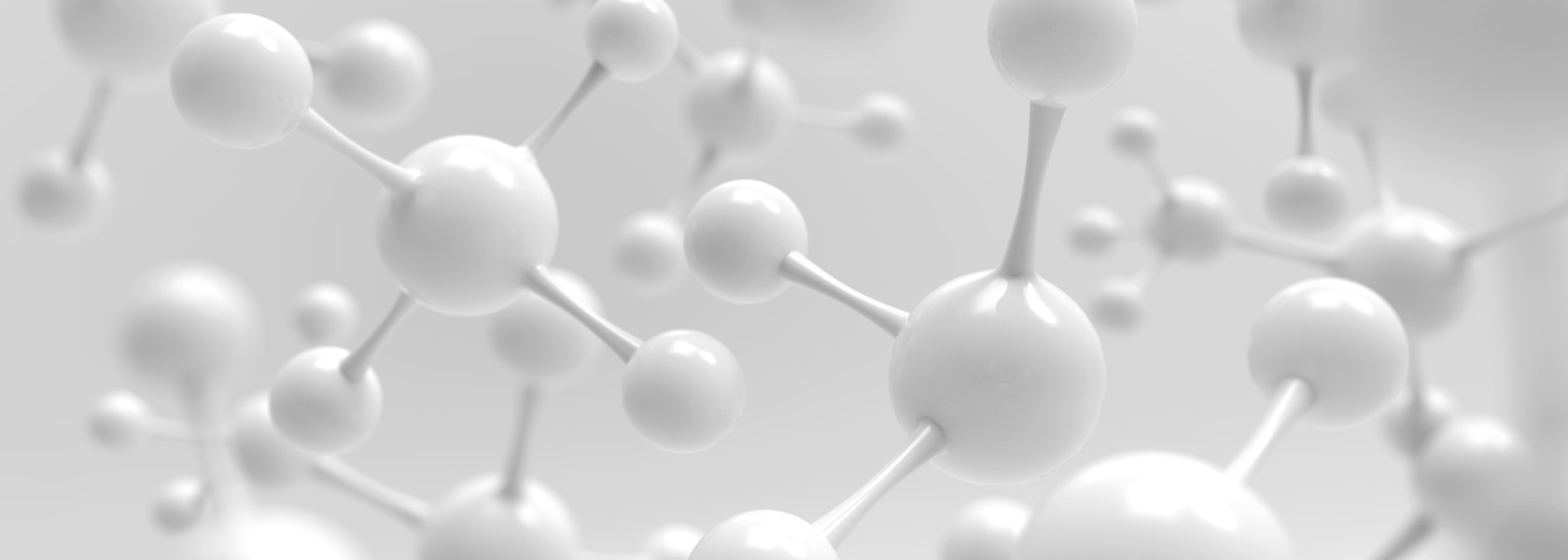